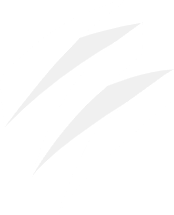
- What we offer
- Who we serve
- Digital Transformation
- Our Approach
- Careers
- About Us
- Contact Us
The World Economic Forum estimates that the world will produce 463 exabytes of data per day – the equivalent of 212,765,957 DVDs.
This jaw-dropping amount of data holds immense potential for the way the finance industry operates and strategizes. Big data analytics is providing solutions that can improve customer experience, operational efficiency, financial models, decision making, stock market insights, and risk management.
As per a 2023 survey, 87% of businesses stated that investing in data and analytics was a high priority.
It is no wonder, then, that as per a 2023 survey, 87% of businesses stated that investing in data and analytics was a high priority. However, a much smaller number – 37% – said that their efforts to improve data quality had proved successful. This highlights one of the ongoing challenges faced by companies, as well as the need for greater data analytics expertise.
In this article, Silverskills will provide an overview of the advantages and challenges associated with big data analytics in finance. By understanding both the advantages and challenges, financial institutions can leverage big data analytics to drive innovation and gain a competitive edge.
Big data analytics is the processing and analysis of vast amounts of data and data sets, called big data, to obtain important insights. It helps companies uncover patterns, trends, and correlations to help them make data-driven decisions.
There are four primary data analysis methods used to determine patterns and uncover insights: descriptive, diagnostic, predictive, and prescriptive.
Big data analytics differs from traditional analytics in the type of data managed as well as the tools used for analysis.
Traditional analytics involves structured data, usually stored in relational databases, and relies on statistical methods and tools such as structured query language (SQL) for querying databases.
Big data analytics deals with vast amounts of data in many formats, including structured, unstructured, and semi-structured. It uses advanced techniques such as data mining and machine learning (ML) to extract information. Often, it requires distributed processing systems to manage the huge volume of data.
Risk Management
Big data analytics is transforming risk management in the finance industry by powering real-time fraud detection, advanced risk assessment models, and stronger compliance monitoring. Combining various data sources, both structured and unstructured, allows for detailed risk profiles, early detection of unusual activities, and proactive risk mitigation, hence boosting financial stability and regulatory compliance.
For example, banks can use predictive analytics models to more accurately assess creditworthiness by analyzing a customer’s financial behavior, spending patterns, and even social media activity. Such dynamic risk assessment is an improvement over traditional methods, which often rely on static financial statements or credit scores.
By identifying risks earlier and more precisely, financial institutions can reduce defaults, optimize capital allocation, and improve overall risk-adjusted returns.
Real-Time Intelligence
One of the most prominent advantages of big data analytics in finance is its ability to provide real-time data. Financial institutions can analyze huge amounts of data as it is generated from numerous sources and in different formats.
This real-time intelligence empowers businesses to swiftly respond to market changes, make quick decisions, and seize opportunities as they arise.
Customer Insights and Personalization
In an increasingly competitive market, personalized customer service has become a key differentiator.
Big data analysis allows financial institutions to gain customer insights through behavior analysis, advanced segmentation, and sentiment tracking. These insights power personalized product recommendations, customized financial solutions, targeted marketing, and enhanced engagement strategies, thus helping to nurture long-term customer relationships and loyalty.
Personalization can also extend to digital interactions. For example, chatbots powered by big data can deliver more relevant and timely responses, enhancing the customer experience. Furthermore, with predictive analytics, financial institutions can anticipate customer needs, such as recommending loan products when clients are likely to make large purchases.
Regulatory Compliance
Regulatory compliance is an increasing concern in the finance sector, with regulations such as the GDPR, Basel III, and the Dodd-Frank Act requiring financial institutions to maintain transparent operations and extensive record-keeping.
Big data analytics helps financial institutions track and store large volumes of regulatory data, ensuring that they remain compliant with current legislation.
Additionally, advanced analytics tools can monitor transactions and flag suspicious activities to meet Anti-Money Laundering (AML) requirements, and can also automate compliance checks, reducing the risk of human error.
Cost Savings
Big data analysis helps reduce costs by identifying ways to make business processes more efficient. By analyzing large datasets, financial institutions can spot unnecessary expenses, streamline operations, and boost productivity.
Furthermore, by streamlining operations, such as underwriting or compliance checks, financial institutions can process more transactions in less time with fewer errors.
Lastly, predictive maintenance of IT systems using big data can prevent expensive downtime or data breaches, while also streamlining core processes such as loan approvals, asset management, and customer onboarding.
Investment and Trading
In investment and trading, big data analytics equips financial professionals with insights for portfolio optimization, algorithmic trading, decision support, and market trend analysis.
Sentiment tracking from news and social media, real-time data analysis, and predictive modeling enable informed investment choices, risk diversification, and agile reactions to market shifts. This improves investment performance and provides a competitive edge in financial markets.
While big data holds great promise for financial institutions, certain challenges need to be overcome to maximize its benefits and ensure effective implementation.
Data Privacy and Security
The finance industry handles vast amounts of highly confidential data, such as customer transactions, investment details, and social security numbers. The integration of big data increases the risk of cyber-attacks, data breaches, and unauthorized access.
The integration of big data increases the risk of cyber-attacks, data breaches, and unauthorized access.
With financial data becoming more digitized, prioritizing data privacy and security is essential. Organizations must comply with complex privacy regulations like the GDPR, DPDPA, PDPL, and other industry-specific standards to protect customer information, prevent data breaches, and maintain customer trust.
Compliance with such regulations can be made more complicated by the need to collect, store, and analyze large datasets from various sources.
Furthermore, ensuring the security of third-party data, such as data from vendors or cloud storage providers, introduces additional vulnerabilities. Implementing robust encryption, secure access controls, and regular auditing are necessary but costly measures.
Regulatory Compliance
While big data analytics can help institutions comply with regulations, it paradoxically also presents challenges regarding compliance.
The financial sector functions under strict regulations covering data usage, storage, sharing, and reporting. Compliance with standards such as Basel III, KYC (Know Your Customer), AML (Anti-Money Laundering) as well as data protection laws demands robust data governance, adherence to reporting requirements, and transparent data management.
Financial institutions, especially smaller ones, may hence find their resources stretched or unable to keep up with regulatory requirements.
High Implementation Costs
Although big data and analytics promise significant benefits, the initial investment can be expensive, particularly for smaller financial institutions.
Costs may include infrastructure setup, data storage, specialized software, and hiring or training personnel with the necessary technical expertise.
Furthermore, the demand for data scientists, machine learning engineers, and cybersecurity experts has surged, making it more difficult for institutions to attract the right talent without significant financial investment.
Lastly, regular investments in hardware and software upgrades are necessary to maintain analytics capabilities. Hence, financial institutions must carefully weigh the potential ROI before embarking on big data projects.
Talent and Skills Gap
There is a shortage of skilled professionals, such as data scientists, analysts, and experts in data management, machine learning, and governance.
This poses a major challenge to implementing and maintaining big data analytics projects. Organizations struggle to recruit, retain, and upskill talent needed to fully leverage technologies and extract actionable insights from complex data.
Infrastructure Integration and Scalability
Big data and analytics demands a scalable, flexible infrastructure that can manage large data volumes, ensure high-speed processing, and support real-time analysis.
Integrating diverse data sources, legacy systems, and new technologies like cloud computing presents challenges, requiring strong data integration strategies, smooth interoperability, and efficient data pipelines to derive valuable insights and make data-driven decisions.
Overcoming these challenges calls for a holistic approach that includes technology investments, talent development, strategic partnerships with regulators, and a robust data governance framework.
The journey towards data-driven operations requires a comprehensive approach. Technological investments must be aligned with changing customer expectations, regulatory requirements, and business objectives. Financial institutions that embrace big data analytics will position themselves as industry leaders in the digital age.
To harness the power of data and analytics in the financial sector, companies must foster a data-centric culture, collaborate with technology providers, and continuously upskill their workforce.
Silverskills is a digital transformation company with over 20 years of experience. We offer big data analytics services, from data lifecycle management to data mining, that help unlock new revenue streams, drive innovation, and enhance customer satisfaction. Contact us now to speak to a data analytics expert.
Please fill the details below. A representative will contact you shortly after receiving your request.